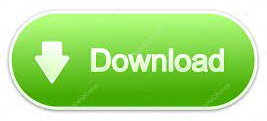
#WTFact Videos In #WTFact Britannica shares some of the most bizarre facts we can find.
Britannica Classics Check out these retro videos from Encyclopedia Britannica’s archives. Thus, should you be looking for a new future discussion point, my query to you then might be some pearls on the nature of a qualifying heteroscedastic funnel shape: How severe must it be? Is there a quantifiable magnitude to said severity, and if so, how would one quantify this and/or what numeric outputs in common statistical software would best support or deny a suspicion based on graphical interpretation? What directions can the funnel point are only some directions suggestive, whereby others are not? Thanks for entertaining my comment, and, as always, thanks for doing what you do. It seemed to me that my resulting plot of a 5 predictor variable regression model featured an obtuse triangular left point that violated homoscedasticity my professors disagreed, stating the triangular “funnel” aspect would be more prominent and overt. Specifically, I was looking for information on how pronounced the triangular/funnel shape must be–and in what directions it may point–to suggest heteroscedasticity in a regression scatterplot of standardized residuals vs standardized predicted values. I’m a fan of your admirable gift to make complicated topics approachable and digestible. Jim, as if by serendipity: having been on your mailing list for years, I looked up your information on multiple regression this weekend for a grad school advanced statistics case study.
I’ve written an article about that discusses these issues in more detail, read Confounding Variables Can Bias Your Results. The strength of the correlation between the potential IV and the DV also plays a role. However, it’s not a simple as a single criterial like that. Instead, I’d look at the strength of the correlations between IVs. You can have low p-values for weak correlation in some cases. Personally, I wouldn’t use p-values to make this determination. I’ve never heard of a formalized rule such as the one that you mention. However, when you’re performing a true, randomized experiment, this type of bias becomes a non-issue. Observational studies are particularly susceptible to this type of omitted variable bias. In this scenario, the degree of bias depends on the strengths of the correlations involved. When you have a potential independent variable that correlates with other IVs and it also correlates with the dependent variable, it becomes a confounding variable and omitting it from the model can cause a bias in the variables that you do include. In some cases, it’s absolutely crucial to include covariates that correlate with other independent variables, although it’s not a sufficient reason by itself.